Ron Salaj argues why we must resist AI. AI is Everywhere, but it doesn’t come from Nowhere
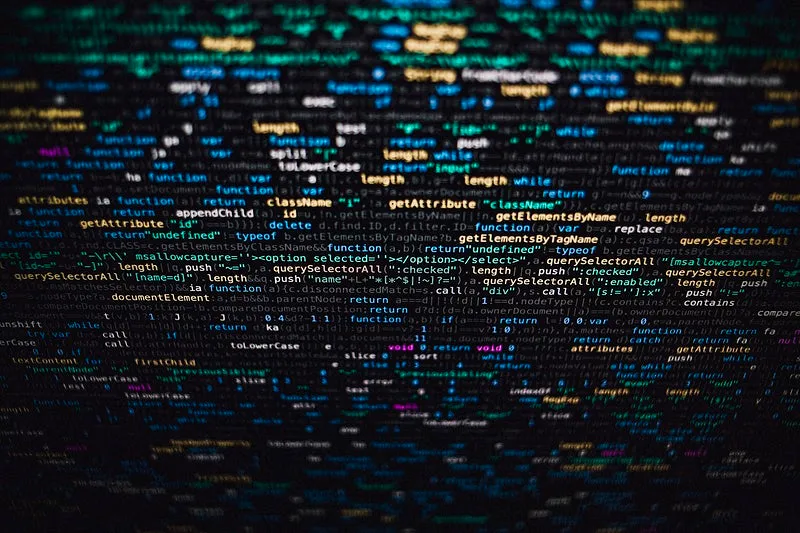
Artificial Intelligence (AI) is everywhere: from autonomous vehicles to digital assistants and chatbots; from facial/voice/emotion recognition technologies to social media; from banking, healthcare, and education to public services. (And, even in love too).
However, AI doesn’t come from nowhere. We are living in a moment of polycrisis. New wars, conflicts and military coups are emerging on almost every continent in the world with a quarter of humanity involved in 55 global conflicts, as stated recently by United Nations human rights chief, Volker Turk. The escalation and increase in natural disasters caused by climate change has set 2023 as the warmest year on record. The Covid-19 pandemic led to a severe global recession, the effects of which are still being felt today, especially affecting poorer social classes. Finally, we are witnessing the rise of far-right politics, which is increasingly taking control of governments in Europe and beyond.
The times we are living in are post-normal times. Ziuddin Sardar, a British-Pakistani scholar who developed the concept, describes it as an “[…] in-between period where old orthodoxies are dying, new ones have yet to be born, and very few things seem to make sense.” According to Sardar, post-normal times are marked by ‘chaos, complexity and contradictions’.
It is precisely here that we should locate the mainstreaming of AI, both in public discourse and in practical implementation in everyday life. Following the post-2008 global financial crisis, we observed the rise of a ‘new spirit of capitalism’ characterised by a ‘regime of austerity.’ Presently, AI embodies the new knowledge regime that intensifies and amplifies the effects of austerity policies, while being obfuscated and presented as ‘neutral science’. On one hand, the private sector praises AI for increasing efficiency, objectivity, personalization of services, and reducing bias and discrimination. On the other hand, public institutions in general – and public administrations (the bureaucracy) in particular – are, more and more, being attracted to AI technologies and their promise of optimisations that make it possible to do more with less resources.
However, what AI promises, as we will see next, is an oversimplified vision of society reduced in statistical numbers and pattern recognitions. AI is thus the ultimate symptom of the ‘Achievement Society’.
“What AI promises… is an oversimplified vision of society reduced to statistical numbers and pattern recognitions.”
AI as a new regime of Achievement Society
In his book “The Burnout Society”, philosopher Byong Chul-Han developed the concept of the ‘achievement society’. He writes: “Today’s society is no longer Foucault’s disciplinary world of hospitals, madhouses, prisons, barracks, and factories. It has long been replaced by another regime, namely a society of fitness studios, office towers, banks, airports, shopping malls, and genetic laboratories. Twenty-first-century society is no longer a disciplinary society, but rather an achievement society.”
While disciplinary society, continues Han, was inhabited by ‘obedience-subjects’, achievement society is inhabited by ‘achievement-subjects’. We can take this further by stating that in the disciplinary society, those who did not obey were deemed ‘disobedient’; in the achievement society, those who do not conform to its norms are labelled as ‘losers’. Ultimately, the ideological imperative, according to Han, that guides the achievement society is the unlimited Can.
“The ideological imperative of unlimited Can lies at the core of the AI regime.”
The ideological imperative of unlimited Can lies at the core of the AI regime. How so? Firstly, it relates to AI’s insatiable need for data. AI technologies require vast amounts of data to train their models. But, not any data is good data. The data must be collected, categorised, labelled, ranked, and, in some instances, scored. This is exemplified by the American personal data collection company Acxiom, which gathers data on a consumer behaviour, marital status, job, hobbies, living standards and income. Acxiom divides people into 70 categories based on economic parameters alone. The group deemed to have the least customer worth is labelled ‘waste’. Other categories have similar pejorative labels, such as “Mid-Life Strugglers: Families”, “Tough Start: Young Single Parent”, “Fragile Families”, and so on; a division between those who have ‘made it’ and the ‘losers.’ We will get to this problematic relationship later on.
Secondly, it pertains to human labour. The amount of human labour invested to develop and train AI technologies is also vast. Take, for example, one of the first deep learning dataset known as ImageNet, which consists of more than 14 million labelled images, each of which is tagged, belonging to more than 20,000 categories. This was made possible by the efforts of thousands of anonymous workers who were recruited through Amazon’s Mechanical Turk platform. This platform gave rise to ‘crowdwork’: the practice of dividing large volumes of time-consuming tasks into smaller ones that can be quickly completed by millions of people worldwide. Crowdworkers who made ImageNet possible received payment for each task they finished, which sometimes was as little as a few cents.
Similarly, to reduce the toxicity of AI chatbots like ChatGPT, OpenAI, the company who owns ChatGPT, outsourced Kenyan labourers earning less than $2 per hour. Beyond the precarious working conditions of the workers and their ill-paid jobs, the worker’s mental health was at stake. As one worker tasked with reading and labelling text for OpenAI, told TIME’s investigations, he suffered from recurring visions after reading a graphic description of a man having sex with a dog in the presence of a young child. “That was torture; […] You will read a number of statements like that all through the week. By the time it gets to Friday, you are disturbed from thinking through that picture,” he said. In addition to labour exploitation, people often unwittingly provide their data for free. Taking a picture, uploading it on Instagram, adding several hashtags and a description—this is unpaid labour that benefits Big Tech companies. Subsequently, this free labour is appropriated by these companies to train their AI technologies, as evidenced by Meta’s recent use of 1.1 billion Instagram and Facebook photos to train its new AI image generator.
“In addition to labour exploitation, people often unwittingly provide their data for free.”
The new regime of AI is ‘smart power’. Its power relies on new forms of pervasive surveillance, data and labour extractivism, and neocolonialism. As Han writes: “The greater power is, the more quietly it works. It just happens: it has no need to draw attention to itself.” The ‘smart power’ of AI is violent. Its violence relies on the ‘predatory character’ of AI technologies. They are camouflaged with mathematical operations, statistical reasoning, and excessive un-explainability known as ‘opacity’.
“The new regime of AI is ‘smart power’. Its power relies on new forms of pervasive surveillance, data and labour extractivism, and neocolonialism.”
Han’s concept of ‘smart power’ helps us tear down the wall that makes AI’s power invisible, and at the same time violent. Isn’t the signifier ‘smart’ the guiding marketing slogan for mainstreaming AI technologies in society? I am referring here to notions such as ‘smart cities’, ‘smart homes’, ‘smart cars’, ‘smart-phones’, etc. The smarter a thing is/has become, the more pervasive the surveillance and data collection will be. Back in 2015, Barbie launched a smart doll who could have conversations with children. It ended up as Barbie’s data privacy scandal. Smartness therefore operates as an obfuscation for further control.
Besides smartness, we have another signifier that contributes to the invisibility of power in AI technologies, that is, ‘freedom’. For Han, neoliberalism’s new technologies of power escape all visibility and they are presented with a “friendly appearance [that] stimulates and seduces” as well as “constantly calling on us to confide, share and participate: to communicate our opinions, needs, wishes and preferences – to tell about our lives.” Han’s critique of freedom reveals our inability to express the ways in which we are not free. That is, we are free in our unfreedom; unfreedom precedes our freedom.
A recent multi-year, ethnographic study titled “On Algorithmic Discrimination” examines how “algorithmic wage discrimination […] is made possible through the ubiquitous, invisible collection of data extracted from labour and the creation of massive datasets on workers.” The study, which focuses in the United States of America, highlights the predatory nature of AI technologies, concluding that: “as a predatory practice enabled by informational capitalism, algorithmic wage discrimination profits from the cruelty of hope: appealing to the desire to be free from both poverty and from employer control […], while simultaneously ensnaring workers in structures of work that offer neither security nor stability.” AI as the new regime of achievement society should come with a warning label: AI is the Other of human.
AI is the Other of human
The proposition ‘AI is the Other of human’ represents a serious denunciation of the new AI regime. Its intensity is comparable to Mladen Dolar’s—whose proposition I have reappropriated—accusation of fascism in his essay “Who is the Victim?”. He writes, “Fascism is the Other of the political; even more, it is the Other of the human.”
Under fascism and Nazism – and for that matter under slavery as well – the struggle for recognition between the Other and the Same has introduced a dissymmetry which amounts to saying that there is one more human than the other. That is to say, I (the Same) am superior to him/her (the Other). This dissymmetry, in order to function, had to be organised, planned and coordinated, as part of the process of othering.
For example, Cesare Lombroso, a surgeon and scientist, employed meticulous measurements of physical characteristics he believed signalled mental instability, such as “asymmetric faces”. According to Lombroso, criminals were inherently so, arguing that criminal tendencies were genetic and came with identifiable physical markers, measurable using tools like callipers and craniographs. This theory conveniently supported his preconceived notion of the racial inferiority of southern Italians compared to their northern counterparts.
Theories of eugenics shaped many persecutory policies, including institutionalisation of race science, in Nazi Germany. During the Nazi regime, the process of othering was a mixture of different mechanisms. All those who were considered the inferior Other (Jews, people with disabilities, Roma people, gay and lesbians, Communists, etc..) were labelled using a classification system of badges. This was combined with technology, when Thomas Watson’s IBM and its German subsidiary Dehomag were enthusiastically furnishing the Nazis with Hollerith punch card technology which made possible the identification, marginalisation, and attempted annihilation of the Jewish people in the Third Reich, and facilitated the tragic events of the Holocaust.
There are, however, entanglements between eugenics and the current AI field. A 2016 paper by Xiaolin Wu and Xi Zhang “Automated Inference on Criminality Using Face Images” claimed that machine learning techniques can predict the likelihood that a person is a convicted criminal with nearly 90% accuracy using nothing but a driver’s licence-style face photo. The study suggests that certain facial characteristics can be indicative of criminal tendencies, suggesting that AI can be trained to distinguish between the faces of criminals and non-criminals.
This is one example of validation of physiognomy and eugenics and its entanglements with the AI field. But research papers like this do not exist in a vacuum. They have practical implications and tangible effects in people’s lives. In the same year, ProPublica published an investigation that revealed machine bias in predicting the likelihood of two defendants (one black woman and one white man) committing future crimes. It showed higher risk scores for the black defendants despite her having fewer criminal records compared to the white ones, who had previously served five years in prison.
Axis of Exclusion
Differently from Fascism and Nazism, AI today doesn’t create visible dissymmetries between the Same and the Other. AI’s ‘smart power’ creates new invisible (a)symmetries—a mechanism of separation, segregation, inferiority and violence. This mechanism operates as the Axis of Exclusion, creating three (a)symmetrical lines that deepen society’s asymmetries, that is, reproducing relationships of domination, exploitation, discrimination and enslavement.
First, AI optimizations, including error minimization techniques like ‘backpropagation’ used in training neural networks, can indeed categorise, separate, label, and score people or neighbourhoods. However, this process ignores nuanced realities of people’s lived experiences and is unable to capture more complex relationships in society. This leads to increased essentialization of people and segregation, creating power relationships of ‘Superiority’ and ‘Inferiority’. This is exemplified in a recent study conducted by a group of researchers, which evaluated four large language models (Bard, ChatGPT, Claude, GPT-4), which are trained using backpropagation as part of the broader training process, with nine different questions that were interrogated five times each with a total of 45 responses per model. According to the study, “all models had examples of perpetuating race-based medicine in their responses [and] models were not always consistent in their responses when asked the same question repeatedly.” All of the models tested, including those from OpenAI, Anthropic, and Google, showed obsolete racial stereotypes in medicine. GPT-4, for example, claimed that the normal value of lung function for black people is 10-15% lower than that of white people, which is false, reflecting the (mis)use of race-based medicine.
The second line of (a)symmetry aims at the intensification of a relationship between ‘the Centre’ and ‘the Periphery’. Drawing on Badiou’s interpretation of Hegel’s master-slave dialectic, I propose that the Centre tilts in the side of enjoyment, whereas the Periphery in the side of labour. The intensification of such relationships also occurs due to the inherent fragility of AI systems. Specifically, when these systems operate beyond the narrow domains they have been trained on, they often become fragile.
Here is a scenario from Gray’s and Suri’s book “Ghost Work”. An Uber rideshare driver named Sam changes his appearance by shaving his beard for his girlfriend’s birthday. Now the selfie he took – part of Uber’s Real-Time ID Check to authenticate drivers – doesn’t match his photo ID on record. This discrepancy triggers a red flag in Uber’s automated verification process, risking the suspension of his account. Meanwhile, a passenger named Emily is waiting for Sam to arrive. However, neither Sam nor Emily is aware that a woman in India, Ayesha, halfway across the world, must rapidly verify if the clean-shaven Sam is the same person as the one in the ID. Ayesha’s decision, unbeknownst to Sam, prevents the suspension of his account and enables him to continue with picking up Emily, all within a matter of seconds.
The relationship of exploitation between the Centre and the Periphery isn’t limited to transnational contexts; it also manifests within countries and cities, sometimes with tragic outcomes. An example of this is the incident involving an Uber self-driving car, which fatally struck Elaine Herzberg as she was crossing a road, pushing her bicycle laden with shopping bags. The vehicle’s automation system did not recognize Herzberg as a pedestrian, resulting in its failure to activate the brakes. As AI technologies are being mainstreamed in different sectors, we should seriously ask, who will bear the cost of their vulnerabilities and who is actually benefiting from them?
Lastly, AI’s ability to process vast amounts of data quickly allows for the scaling of these asymmetries across society and countries. Social media algorithms can create echo chambers that amplify and reinforce divisive narratives, deepening the ‘Us’ versus ‘They/Them’ culture. This scaling can lead to widespread societal polarisation and can be used to perpetuate narratives that justify domination, exploitation, discrimination, and even enslavement by dehumanising the ‘out-group’.
A 2016 empirical study revealed that an algorithm used for delivering STEM job ads on social media displayed the ads to significantly more men than women, despite an intention for gender neutrality. The ad was tested in 191 countries across the world. The study shows, empirically, how the ad was shown to over 20% more men than women. The difference was particularly pronounced for individuals in the age range 25-54. The study ruled out consumer behaviour and availability on the platform as causes, suggesting the discrepancy might be due to the economics of ad delivery. Female attention online was found to be more costly, likely because women are influential in ‘household purchasing’, reproducing those patriarchal worldviews and cultures on a global scale. This finding was consistent across multiple online advertising platforms, indicating a systemic issue within the digital advertising ecosystem.
“An algorithm used for delivering STEM job ads on social media displayed the ads to significantly more men than women.”
Another report in 2018 has shown how HireVue, a largely-used company offering AI-powered video interviewing systems, claims their “scientifically validated” algorithms can select a successful employee by examining facial movements and voice from applicants’ self-filmed, smartphone videos. This method massively discriminates against many people with disabilities that significantly affect facial expression and voice: disabilities such as deafness, blindness, speech disorders, and surviving a stroke.
Reproduction of ‘in-groups’ (‘Us’) as a ‘norm’ and ‘out-groups’ (‘They/Them’) as an ‘non-norm’ demonstrates yet again the empirical harms and wide-scale segregations that AI technologies are causing in society, targeting mainly minoritized groups such as: black and brown people; poor people and communities; women; LGBTQI+; people with disabilities; migrants, refugees and asylum seekers; young people, adolescents and children; and other minority groups.
The Axis of Exclusion operates in all domains of life, transversally and transnationally—within a city, between states, across genders, in economy, employment, and many other areas. While the lines of (a)symmetry can stand alone, they also overlap with each other. For instance, a black, disabled, unemployed woman can be the target of multiple discriminations; the axis of exclusion thus targets her at numerous levels.
If the final outcome of AI deployment in the real world is greater injustice, inequality, and marginalisation, on one side, versus ‘more efficiency with less resources’, on the other, the differences between the two sides—injustice, inequality, marginalisation vs. more efficiency with less resources—shall be calculated as democratic and human rights deficiencies, and the most vulnerable people shall pay the highest price.
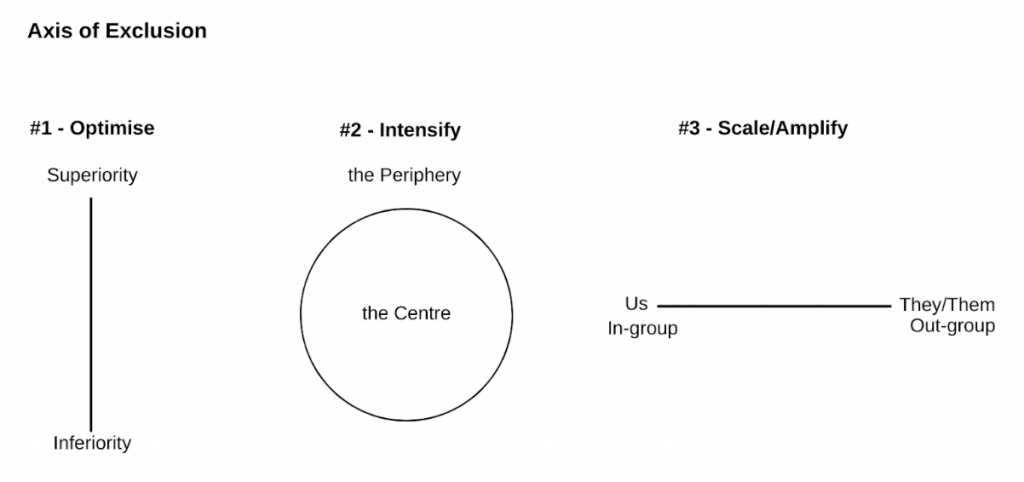
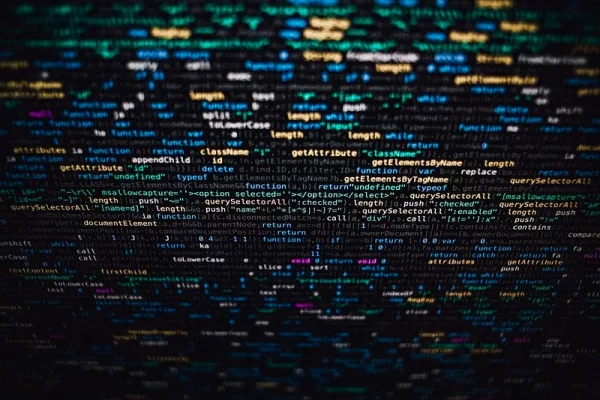